By Nirmal John
A Complete Guide to Understanding and Leveraging Data
Tuesday April 1, 2025
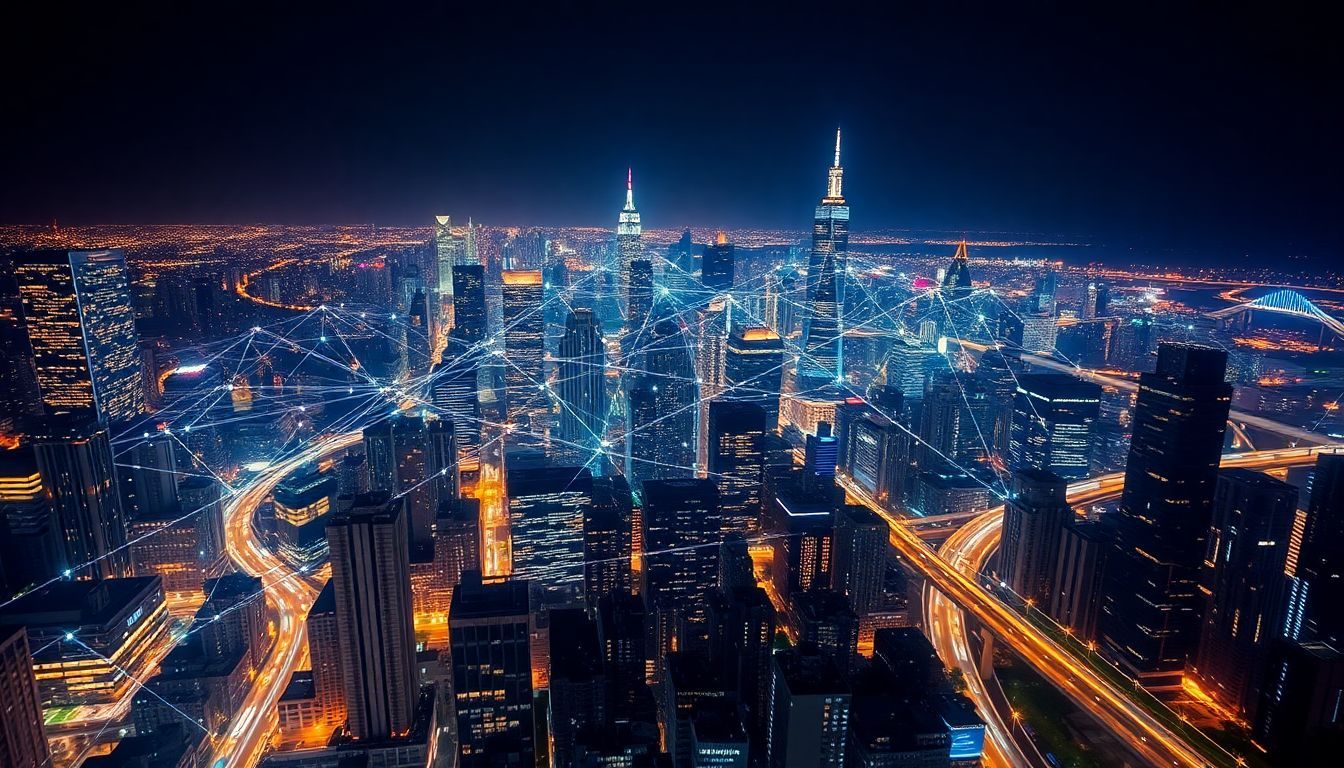
A Complete Guide to Understanding and Leveraging Data
Ever wonder how Netflix always knows what shows you’ll love? Or how your bank spots fraud before it happens? The answer lies in data analytics. It’s everywhere, transforming how businesses operate and how we live our daily lives. According to IBM, the world creates approximately 2.5 quintillion bytes of information every day—that’s 2.5 followed by 18 zeros! This massive volume presents both challenges and opportunities for organizations worldwide.
In this comprehensive guide, we’ll explore what makes advanced analytics powerful, why it matters, the technologies that power it, and how various industries are using these insights to drive innovation and growth. Whether you’re a business leader, technology professional, or simply curious about the data revolution reshaping our world, this article will provide valuable insights into one of the most transformative technological developments of our time.
The Foundation of Modern Analytics Systems
To leverage the power of information effectively, we must first understand what makes it different from traditional processing and analytics approaches.
What Makes Data-Driven Systems Different
Advanced analytics is far more than just a large collection of information. It refers to enormously complex and rapidly growing data sets that traditional processing applications cannot effectively manage. These massive volumes of both structured and unstructured information require specialized approaches to storage, processing, and analysis.
According to a Gartner definition, modern analytics systems are characterized by high volume, high velocity, and high variety. This definition has since expanded to include two additional dimensions: veracity and value. Together, these “5 V’s” provide a framework for understanding what makes information management uniquely challenging in today’s landscape.
Understanding the Five Key Dimensions
These five defining characteristics help clarify what sets modern information systems apart from conventional management methods:
Volume and Scale
This refers to the sheer magnitude of information being generated and collected. We’re talking about terabytes (1,000 gigabytes), petabytes (1,000 terabytes), or even exabytes (1,000 petabytes) of information. To put this in perspective, one petabyte could store approximately 20 million filing cabinets of text. According to Facebook’s statistics, Social media platforms generate enormous volumes—Facebook users share approximately 100 million photos and videos daily.
Processing Speed
The velocity at which information is created, accumulated, and processed has accelerated dramatically. Much of today’s data requires real-time or near-real-time processing and analysis. Stock market trades, website clicks, mobile app interactions, and IoT sensor readings all generate streams that flow continuously. Therefore, organizations must develop systems capable of instantaneously ingesting and analyzing information to derive timely insights.
Information Diversity
Modern information comes in numerous formats and types—structured (databases, spreadsheets), semi-structured (JSON, XML), and unstructured (images, videos, social media posts, text documents). This diversity creates challenges for traditional database systems designed primarily for structured data. According to IDC research, over 80% of enterprise information is now unstructured, highlighting the importance of technologies that can process and analyze diverse types.
Data Quality and Reliability
This dimension addresses the reliability and trustworthiness of information. With so much coming from various sources, ensuring accuracy, consistency, and completeness becomes critical. Poor quality can lead to flawed analysis and misguided decisions. Organizations must implement rigorous governance practices, quality controls, and validation processes to maintain high levels of veracity.
Business Impact
Ultimately, the purpose of collecting and analyzing information is to extract meaningful insights that drive better decision-making and create business value. The challenge lies in identifying the relevant data points within vast sets and transforming them into actionable intelligence. According to McKinsey Global Institute, organizations that leverage analytics effectively can increase their operating margins by up to 60%.
Why Advanced Analytics Matters
Understanding why these systems matter has become essential for organizations across all industries. Analytics enables businesses to make more informed decisions based on evidence rather than intuition. By analyzing large datasets, organizations can uncover hidden patterns, correlations, and trends that would be impossible to detect manually or with traditional tools.
For example, healthcare providers can analyze patient information to identify risk factors and predict disease outbreaks before they become widespread. Retailers can examine customer purchasing patterns to optimize inventory management and personalize marketing campaigns. Financial institutions can monitor transaction data in real-time to detect fraudulent activities and prevent security breaches.
The strategic importance continues to grow as organizations seek competitive advantages in increasingly data-driven markets. According to PwC research, data-driven companies are 3 times more likely to report significant improvements in decision-making compared to those who rely less on analysis.
Technology Ecosystem for Advanced Analytics
Managing and analyzing massive information sets requires specialized technologies and tools designed to handle unique characteristics. These solutions enable organizations to store, process, and derive insights from datasets that would overwhelm traditional systems.
Modern Storage Architecture
The foundation of any analytics initiative is a robust storage infrastructure capable of handling large volumes of diverse information types:
Enterprise Data Warehouses
These specialized databases are optimized for analytics and reporting purposes, primarily handling structured information from operational systems. Data warehouses use a schema-on-write approach, meaning information is transformed and organized according to a predefined schema before storage. This makes them excellent for stable, well-understood requirements and regular reporting needs. Solutions like Snowflake and Amazon Redshift provide cloud-based capabilities with significant scalability.
Flexible Information Repositories
Unlike warehouses, data lakes store information in its raw, native format without requiring transformation before storage (schema-on-read). This approach offers tremendous flexibility, allowing organizations to store structured, semi-structured, and unstructured information in one repository. Solutions like Azure Data Lake or AWS Lake Formation enable scientists and analysts to access and analyze diverse types using various processing tools and techniques.
Cloud-Based Solutions
Cloud platforms have revolutionized storage by offering virtually unlimited scalability, pay-as-you-go pricing models, and global accessibility. Services like Amazon S3, Google Cloud Storage, and Microsoft Azure Blob Storage provide cost-effective solutions for storing massive datasets while ensuring reliability through redundant storage across multiple locations. According to Flexera’s State of the Cloud Report, 94% of enterprises now use cloud services, with many specifically leveraging cloud platforms for advanced applications.
Non-Relational Databases
Traditional relational databases struggle with the volume and variety of modern information needs. NoSQL (Not Only SQL) databases address these limitations with flexible schema designs and horizontal scalability. MongoDB, Cassandra, and HBase are popular solutions that excel at handling unstructured and semi-structured data while maintaining performance at scale.
Processing Frameworks for Complex Analysis
Processing complex datasets requires frameworks designed for distributed computing across clusters of machines:
Distributed Computing Ecosystem
The Apache Hadoop ecosystem revolutionized processing by introducing a framework for distributed storage (HDFS) and processing (MapReduce) across commodity hardware clusters. This enables organizations to process massive datasets by breaking them into smaller chunks and processing them in parallel across many machines. As a result, this approach dramatically reduces processing time while providing built-in redundancy. The ecosystem includes numerous complementary projects like Hive (SQL-like queries), Pig (data flow language), and HBase (NoSQL database).
In-Memory Processing Solutions
Apache Spark has emerged as a faster alternative to Hadoop’s MapReduce, offering in-memory processing capabilities that accelerate operations by up to 100 times. Spark provides a unified platform for batch processing, real-time streaming, machine learning, and graph processing through its components: Spark SQL, Spark Streaming, MLlib, and GraphX. According to the Apache Spark website, over 1,000 organizations across various industries now use it for data processing.
Real-Time Stream Analysis
Apache Flink specializes in stream processing with true event-time processing and exactly-once semantics, making it ideal for applications requiring real-time analytics with guaranteed accuracy. Flink processes information as it arrives rather than in batches, enabling lower latency for time-sensitive applications like fraud detection and network monitoring.
High-Throughput Message Handling
Apache Kafka serves as a distributed streaming platform capable of handling trillions of events per day. It provides a unified, high-throughput, low-latency platform for real-time feeds. Organizations use Kafka to build real-time streaming pipelines that reliably move information between systems and applications, and to build real-time streaming applications that transform or react to data streams.
Visualization and Analysis Tools
After storing and processing information, organizations need tools to analyze and visualize insights:
Interactive Dashboard Solutions
Tableau helps users create interactive dashboards and reports without requiring extensive technical knowledge. It connects to various sources and enables drag-and-drop functionality to build compelling visual representations of complex datasets. According to Tableau’s research, organizations using visual analytics tools are 28% more likely to find timely information compared to those using traditional reporting tools.
Integrated Business Intelligence
Microsoft’s Power BI offers similar visualization capabilities with deep integration into the Microsoft ecosystem. It combines cloud-based analytics services with a desktop application for creating and publishing interactive dashboards and reports. Power BI’s AI capabilities can automatically identify patterns and generate insights from datasets.
Associative Analytics Platforms
Qlik’s associative analytics engine differentiates it from other BI tools by maintaining relationships between all data points. This approach allows users to explore information more intuitively by seeing connections that might be missed in query-based tools. Qlik also offers augmented analytics capabilities powered by AI to suggest insights and automate preparation tasks.
Analytical Programming Environments
Data scientists and analysts often use languages like Python and R with specialized libraries for advanced analytics. Python’s ecosystem includes NumPy and Pandas for manipulation, Scikit-learn for machine learning, and Matplotlib and Seaborn for visualization. These tools provide the flexibility and power needed for sophisticated statistical analysis and predictive modeling.
Real-World Industry Applications
Examining practical applications across industries reveals the transformative potential of advanced analytics. Here’s how different sectors leverage insights to drive innovation and solve complex problems:
Healthcare Transformation Through Analytics
The healthcare industry generates enormous volumes of information from electronic health records (EHRs), medical imaging, wearable devices, genetic sequencing, and clinical trials. By harnessing this effectively, healthcare providers and researchers can improve patient outcomes while reducing costs.
Predictive Health Monitoring
Healthcare organizations analyze population health information to identify risk factors and predict disease outbreaks. For instance, Google’s research team developed models that can predict patient outcomes, including the likelihood of hospital readmission and potential complications, with high accuracy. These predictive capabilities enable proactive interventions that prevent conditions from worsening.
Individualized Treatment Approaches
By analyzing genetic information alongside clinical records, healthcare providers can develop customized treatment plans tailored to individual patients’ genetic profiles. This precision medicine approach improves efficacy while reducing adverse effects. According to the National Institutes of Health, precision medicine initiatives have already led to breakthrough treatments for certain cancers and rare genetic disorders.
Healthcare Operations Enhancement
Hospitals and clinics use analytics to optimize resource allocation, streamline patient flow, and reduce wait times. By analyzing admission patterns, procedure durations, and staff availability, healthcare facilities can better schedule operations and allocate resources where they’re most needed. A Deloitte study found that healthcare organizations using advanced analytics reduced administration costs by up to 15%.
Pharmaceutical Innovation
Pharmaceutical companies leverage analytics to accelerate the drug discovery process. By analyzing chemical compounds, biological interactions, and clinical trial results, researchers can identify promising candidates more efficiently. This data-driven approach has the potential to significantly reduce the time and cost of bringing new medications to market.
Financial Services Evolution
The financial services industry has been at the forefront of analytics adoption, using advanced systems to enhance security, improve customer service, and optimize investment strategies.
Security and Risk Management
Financial institutions analyze transaction patterns in real-time to identify potentially fraudulent activities. Machine learning algorithms trained on historical fraud data can detect anomalies that might indicate unauthorized transactions. According to Experian, these systems can reduce false positives by up to 50% while improving detection rates.
Advanced Credit Assessment
Lenders use alternative data to evaluate creditworthiness more accurately than traditional credit scores alone. By analyzing various sources—including payment histories for utilities and rent, social media behavior, and even smartphone usage patterns—financial institutions can assess risk for customers with limited credit histories. This approach expands financial inclusion while maintaining appropriate risk controls.
Quantitative Trading Strategies
Investment firms employ sophisticated algorithms that analyze market information, news sentiment, social media trends, and other factors to execute trades at optimal prices and times. These high-frequency trading systems can process market information and execute transactions in microseconds, identifying opportunities invisible to human traders.
Custom Financial Products
Banks and insurance companies analyze customer information to offer tailored product recommendations and personalized advice. By understanding customers’ financial situations, goals, and risk tolerances, these institutions can provide more relevant services. According to Accenture research, 91% of consumers are more likely to do business with companies that provide personalized experiences.
Retail Industry Innovations
Retailers collect vast amounts of information from point-of-sale systems, e-commerce platforms, loyalty programs, social media, and in-store sensors. This enables more targeted marketing, efficient operations, and enhanced customer experiences.
Personalized Shopping Experiences
Retailers analyze purchase history, browsing behavior, and demographic information to create detailed customer profiles. These insights drive personalized marketing campaigns and product recommendations. Amazon’s recommendation engine, which generates over 35% of the company’s revenue according to McKinsey, exemplifies the power of personalization based on advanced analytics.
Supply Chain Optimization
By analyzing sales patterns, seasonal trends, and external factors like weather and local events, retailers can optimize inventory levels across locations. This reduces carrying costs while ensuring product availability. Walmart’s supply chain analytics system processes over 2.5 petabytes of information every hour to maintain optimal inventory levels across its global network of stores.
Strategic Pricing Systems
Dynamic pricing strategies powered by analytics enable retailers to adjust prices based on demand, competition, and customer willingness to pay. These systems analyze competitor pricing, historical sales data, and even customer browsing patterns to set optimal prices that maximize both sales volume and profit margins.
Location-Based Retail Analytics
Retailers use geographic and demographic information to optimize store locations, layout, and product assortment. By analyzing foot traffic patterns, local demographics, and competitive landscape, retailers can make better decisions about where to establish new stores and how to configure existing ones for maximum performance.
Manufacturing and Industry 4.0 Advancements
Manufacturing companies are embracing “Industry 4.0″—the fourth industrial revolution characterized by smart factories and connected systems. Advanced analytics plays a central role in this transformation:
Smart Maintenance Systems
Manufacturers use sensor data from equipment to predict when machines will require maintenance, reducing downtime and extending asset lifespans. According to Deloitte, predictive maintenance increases equipment availability by 10-20% while reducing maintenance costs by 5-10%.
Automated Quality Assurance
Advanced analytics combined with computer vision systems can detect defects and quality issues in real-time during production. These systems analyze images and sensor readings to identify anomalies that might indicate problems, enabling immediate corrections before defective products leave the factory.
Integrated Supply Networks
Manufacturers analyze information from suppliers, production facilities, logistics providers, and retailers to optimize their end-to-end supply chains. This holistic view enables better demand forecasting, inventory management, and logistics planning, resulting in cost reductions and improved customer service.
Continuous Production Improvement
By analyzing production data, manufacturers can identify bottlenecks and inefficiencies in their processes. These insights drive continuous improvement initiatives that increase productivity and reduce waste. According to GE Digital, manufacturers implementing data-driven process optimization have achieved productivity improvements of up to 25%.
Advantages and Implementation Challenges
As organizations seek to leverage advanced analytics effectively, they must also recognize the challenges and responsibilities that come with massive information collection and analysis.
Strategic Benefits of Data-Driven Approaches
The strategic application of analytics offers numerous advantages that can transform organizations across industries:
Enhanced Strategic Decision-Making
Data-driven decisions based on comprehensive analysis typically yield better outcomes than those based on intuition or limited information. Organizations with mature analytics capabilities can identify patterns and trends that inform strategic decisions, from product development to market expansion. According to Harvard Business Review, organizations that place data at the center of their decision-making approaches improve their operating profit by 6% compared to competitors.
Operational Excellence
Analytics can identify inefficiencies and optimization opportunities throughout organizational processes. Whether streamlining manufacturing operations, reducing energy consumption, or optimizing logistics routes, data-driven insights lead to significant cost savings and productivity improvements. UPS, for example, saves an estimated 10 million gallons of fuel annually by using analytics to optimize delivery routes.
Experience Personalization
Understanding customer preferences, behaviors, and needs at a granular level enables organizations to deliver highly personalized experiences. This personalization increases customer satisfaction, loyalty, and lifetime value. Netflix estimates that its recommendation system, powered by analytics, saves the company $1 billion annually through improved customer retention.
Market-Driven Innovation
Analytics can reveal unmet customer needs and market opportunities that drive innovation. By analyzing customer feedback, usage patterns, and market trends, organizations can develop products and services that better address customer requirements. According to PwC’s Innovation Benchmark report, companies that leverage analytics in their innovation processes are 19% more likely to report above-average profitability.
Proactive Risk Mitigation
Comprehensive analysis helps organizations identify potential risks before they materialize. From credit risk in financial services to safety risks in manufacturing, predictive analytics enables proactive risk management strategies that reduce exposure and mitigate impacts when incidents do occur.
Implementation Hurdles and Considerations
Despite its benefits, implementing analytics initiatives comes with significant challenges that organizations must address:
Information Security and Privacy
As organizations collect more personal data, they face increasing responsibility to protect it from breaches and misuse. Regulatory frameworks like the European Union’s General Data Protection Regulation (GDPR) and the California Consumer Privacy Act (CCPA) impose strict requirements on collection, storage, and processing. According to IBM’s Cost of a Data Breach Report, the average cost of a breach in 2023 reached $4.45 million, highlighting the financial risks of inadequate security.
Information Quality Management
The value of insights derived from analytics depends entirely on the quality of the underlying information. Poor quality—resulting from inaccuracies, incompleteness, or inconsistencies—can lead to flawed analyses and misguided decisions. Organizations must implement robust governance frameworks and quality control processes to ensure reliable inputs for their analytics pipelines.
Technical Infrastructure Requirements
Implementing analytics solutions requires specialized infrastructure, software, and technical expertise. Organizations must invest in storage systems, processing frameworks, analysis tools, and the network infrastructure to support them. According to Gartner, the technical complexity of implementations remains a significant barrier to adoption for many organizations.
Analytical Talent Acquisition
The demand for data scientists, engineers, and analysts far exceeds the available supply of qualified professionals. Organizations struggle to recruit and retain talent with the specialized skills needed to implement and maintain advanced systems. According to IBM, the number of jobs for data professionals will increase by 28% by 2026, creating an even wider talent gap.
Responsible Analysis Practices
The use of advanced analytics raises important ethical questions about surveillance, algorithmic bias, and automated decision-making. Organizations must consider the ethical implications of their practices and ensure that their analytics processes don’t perpetuate discrimination or unfair treatment. Transparency and accountability in usage are becoming increasingly important to maintain public trust.
Building Your Analytics Capability
For organizations looking to leverage analytics for strategic advantage, a structured approach to implementation is essential. Here’s how to begin the journey:
Strategic Needs Assessment
A successful analytics initiative starts with a clear understanding of the business problems you’re trying to solve or the opportunities you want to exploit:
Business Challenge Identification
Begin by identifying specific business challenges that could benefit from data-driven insights. These might include customer churn, operational inefficiencies, product quality issues, or missed market opportunities. Prioritize problems based on their potential business impact and the availability of relevant information.
Value Proposition Development
Develop detailed use cases that define how analytics will address your identified problems. Specify the information sources required, the analysis methods to be employed, and the expected outputs and actions. Start with use cases that offer quick wins to build momentum and demonstrate value.
Investment Return Analysis
Estimate the return on investment for your analytics initiatives by quantifying potential benefits—such as increased revenue, cost reductions, or risk mitigation—and comparing them to implementation costs. According to MIT Sloan Management Review, organizations with the most mature analytics capabilities are twice as likely to report high competitive performance.
Information Resource Assessment
Catalog the information assets currently available within your organization and identify gaps that might need to be filled through additional collection or external sources. This inventory should include types, volumes, update frequencies, and quality assessments.
Analytics Team Construction
Assembling the right team with complementary skills is crucial for success:
Analytical Expertise
These professionals combine statistical expertise, programming skills, and domain knowledge to extract insights from complex datasets. They design experiments, build predictive models, and translate analysis results into business recommendations.
Technical Foundation Builders
Data engineers build and maintain the infrastructure required for storing, processing, and analyzing information. They design pipelines, implement ETL (Extract, Transform, Load) processes, and ensure quality and availability.
Business Translation Specialists
These team members focus on analyzing information to answer specific business questions. They create reports and visualizations that communicate insights to stakeholders and support decision-making processes.
Subject Matter Specialists
These experts provide essential context for interpretation. Their understanding of business processes, customer behaviors, and market dynamics helps ensure that analytics efforts address relevant problems and produce actionable insights.
Leadership and Direction
This leadership role establishes the strategic direction for data initiatives, ensures alignment with business objectives, and advocates for data-driven decision-making throughout the organization.
Implementation Roadmap Development
A comprehensive strategy guides your organization’s approach to implementation:
Technology Selection Process
Choose appropriate technologies for storage, processing, analytics, and visualization based on your specific requirements. Consider factors like volume and velocity, required processing speed, integration with existing systems, and total cost of ownership.
Information Management Framework
Establish policies, procedures, and standards for management throughout its lifecycle. This framework should address quality, security, privacy, compliance, and accessibility considerations.
Initial Proof of Concept
Begin with a small-scale pilot project to test your approach, identify challenges, and demonstrate value. Choose a project with manageable scope but sufficient impact to gain organizational support for broader initiatives.
Enterprise-Wide Expansion
Once pilot projects prove successful, scale your analytics capabilities across the organization. Integrate systems with existing business applications and processes to maximize value and drive adoption.
Iterative Improvement Cycles
Establish mechanisms for measuring the effectiveness of your initiatives and identifying opportunities for improvement. Regularly review and refine your collection, analysis methods, and decision-making processes based on outcomes and evolving business needs.
Future-Ready Analytics Strategy
Understanding how to leverage information effectively has become essential for organizations seeking to thrive in today’s data-driven business environment. The ability to collect, process, and analyze massive datasets unlocks insights that drive innovation, improve efficiency, enhance customer experiences, and create competitive advantages.
While implementing analytics initiatives presents significant challenges—from technical complexity and skills shortages to privacy concerns and ethical considerations—the potential benefits far outweigh these difficulties for organizations that approach implementation strategically.
As information volumes continue to grow exponentially and technologies become increasingly sophisticated, the gap between organizations that effectively leverage analytics and those that don’t will likely widen. By taking a thoughtful, structured approach to implementation—starting with clear business objectives, assembling the right team, selecting appropriate technologies, and establishing robust governance practices—organizations can position themselves for success in the data-driven future.
The journey to analytics maturity is continuous, requiring ongoing investment, adaptation, and learning. However, organizations that commit to this journey will find themselves better equipped to anticipate market changes, respond to customer needs, optimize operations, and identify new opportunities in an increasingly complex and dynamic business environment.
About the author
Recent articles
Quad Core Dedicated Servers
Interested in Quad Core Dedicated Servers? View our inventory. What is a quad core dedicated...
Read More8 Core Dedicated Servers
For website owners looking to eliminate their hardware bottlenecks, the massive power capabilities of an...
Read MoreHow Unmetered Servers Can Help Businesses Grow
If you have a business website that is growing and expanding, it is essential that...
Read More