By Nirmal John
AI Classification Training Models: A Comprehensive Guide with Examples
Tuesday April 1, 2025
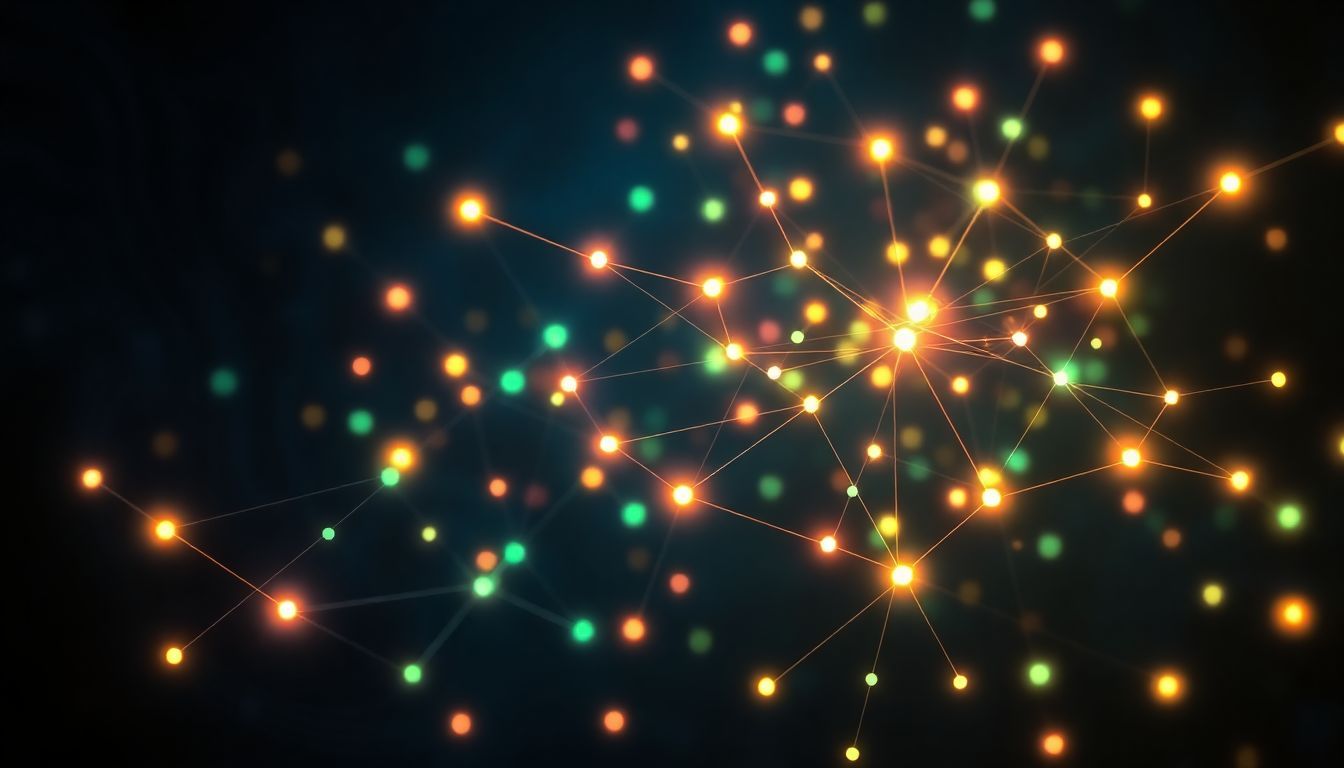
AI Classification Training Models: A Comprehensive Guide with Examples
Imagine a doctor using AI to instantly detect cancer from an X-ray. This is the powerful reality of AI classification training models in action. These sophisticated systems are revolutionizing how businesses and industries make critical decisions across healthcare, finance, retail, and more. This comprehensive guide explores the world of AI classification models, providing practical examples of how they’re implemented in different sectors, and offering insights on selecting the right model for your specific needs.
Understanding AI Classification: Core Concepts and Frameworks
AI classification stands as a foundational concept in machine learning and artificial intelligence. Before diving into specific models, it’s essential to understand the fundamental principles that drive these systems.
What is AI Classification?
AI classification involves teaching computers to systematically categorize data points into predefined classes or categories. Much like teaching a child to distinguish between cats and dogs, AI classification employs supervised learning techniques where algorithms learn from labeled examples. The system analyzes patterns and features in the training data to develop a model that can accurately assign new, unseen data points to the correct category.
Classification differs from other machine learning approaches like regression (which predicts continuous values) or clustering (which groups similar items without predefined categories). The classification process involves feature extraction, model training, and validation – all critical steps to ensure reliable results when the model encounters new data in real-world applications.
Types of Classification Tasks in Modern AI Systems
Classification tasks come in several distinct varieties, each suited to different applications:
Binary classification involves distinguishing between two classes, like determining whether an email is “spam” or “not spam.” This foundational approach powers many everyday applications, from fraud detection systems to medical diagnostics.
Multi-class classification handles scenarios with more than two categories. For example, an image recognition system might categorize fruits as apples, oranges, bananas, or grapes. Voice assistants use multi-class classification to identify different commands or requests.
Multi-label classification represents more complex scenarios where items can simultaneously belong to multiple categories. A news article might be classified under both “politics” and “economy,” or a movie might receive tags for “action,” “sci-fi,” and “thriller” simultaneously. This approach is particularly valuable for content recommendation systems and complex diagnostic tools.
Key Metrics for Evaluating Classification Models Effectively
Rigorous evaluation is essential for developing reliable classification systems. Several complementary metrics help assess different aspects of model performance:
Accuracy provides a straightforward measure of overall correctness – the percentage of predictions the model gets right. While intuitive, accuracy can be misleading with imbalanced datasets where one class appears much more frequently than others.
Precision measures how many of the predicted positives are actually positive, highlighting a model’s ability to avoid false positives. This metric is crucial in applications where false positives are costly, such as spam filtering or fraud detection.
Recall (also called sensitivity) measures how many of the actual positives the model correctly identified, reflecting its ability to find all relevant instances. High recall is essential in medical screening where missing a positive case could have serious consequences.
F1-score balances precision and recall through their harmonic mean, providing a single metric particularly useful when classes are imbalanced or when both false positives and false negatives carry significant costs.
AUC-ROC (Area Under the Receiver Operating Characteristic curve) measures the model’s ability to distinguish between classes across different threshold settings, offering a comprehensive view of performance regardless of the specific classification threshold chosen.
Each of these metrics serves a distinct purpose in the evaluation process, and the appropriate choice depends on the specific requirements and constraints of your application.
Popular AI Classification Training Models: Strengths and Applications
The field offers numerous classification models, each with unique characteristics. Understanding their strengths and limitations helps in selecting the right approach for specific problems.
Logistic Regression: Simplicity and Interpretability
Logistic regression serves as an excellent starting point for many classification tasks despite its deceptively simple name. Unlike linear regression, it predicts the probability of a binary outcome using a logistic function that transforms continuous values into a range between 0 and 1.
Its key advantages include:
- Computational efficiency requiring minimal processing power
- High interpretability where each feature’s contribution to the prediction is clear
- Strong performance on linearly separable data
- Natural probability outputs that can be used for decision thresholds
However, logistic regression struggles with complex, non-linear relationships and can’t capture intricate patterns without manual feature engineering. Despite these limitations, it remains widely used in medical risk assessment, marketing conversion prediction, and preliminary fraud detection systems.
Support Vector Machines (SVM): Finding Optimal Boundaries
Support Vector Machines excel at creating clear decision boundaries between classes, even in high-dimensional spaces. The “kernel trick” – a mathematical technique that implicitly maps data into higher dimensions – enables SVMs to handle non-linear classification tasks efficiently.
SVMs are particularly valuable when:
- Working with clear margins of separation between classes
- Dealing with high-dimensional data like text classification
- Operating in domains where decision boundary precision is crucial
- Working with limited training examples
Real-world applications include sentiment analysis, image classification, and bioinformatics. For example, SVMs have been successfully implemented in protein classification and handwriting recognition systems where their ability to maximize the margin between classes improves generalization to new data.
Decision Trees: Transparent Decision-Making Processes
Decision trees create a flowchart-like structure of decision points, making them among the most intuitive classification models. Each node represents a feature, each branch a decision rule, and each leaf a classification outcome.
Their strengths include:
- Natural visualization capabilities that non-technical stakeholders can understand
- Automatic feature selection as the algorithm identifies the most informative variables
- Handling of both numerical and categorical data without preprocessing
- Minimal assumption requirements about the underlying data distribution
Decision trees find applications in customer segmentation, medical diagnosis protocols, and financial risk assessment. Their transparency makes them particularly valuable in regulated industries where model decisions must be explainable to authorities or customers.
Random Forest: Harnessing Collective Intelligence
Random forests leverage the power of ensemble learning by combining multiple decision trees to produce a more accurate and stable model. Each tree in the forest is trained on a random subset of the data and features, reducing the risk of overfitting through diversity.
This approach offers several advantages:
- Significantly improved accuracy compared to single decision trees
- Built-in protection against overfitting through aggregation of diverse trees
- Ability to handle thousands of input variables without deletion
- Automatic generation of feature importance rankings
Random forests power recommendation systems, land cover classification from satellite imagery, and financial market prediction tools. Their robustness makes them suitable for applications where reliability across diverse conditions is essential.
Neural Networks: Deep Learning for Complex Patterns
Neural networks, particularly deep learning architectures, have revolutionized classification performance for complex data types. These models consist of interconnected layers of nodes (neurons) that can learn intricate patterns through iterative optimization.
Key neural network architectures include:
Multi-Layer Perceptrons (MLPs) – The foundational neural network architecture with fully connected layers, suitable for tabular data and basic pattern recognition.
Convolutional Neural Networks (CNNs) – Specialized for grid-like data such as images, using convolutional filters to detect spatial patterns regardless of their position.
Recurrent Neural Networks (RNNs) – Designed for sequential data like text or time series, maintaining an internal memory to process sequences of inputs.
Transformer Models – The newest generation of neural networks excelling at language tasks through attention mechanisms that capture contextual relationships.
While powerful, neural networks typically require substantial training data, computational resources, and expertise to implement effectively. They’ve transformed image recognition, natural language processing, and speech recognition systems.
Real-World Examples of AI Classification in Action
AI classification training models power innovative solutions across numerous industries. These practical implementations demonstrate how the theoretical concepts translate into tangible benefits.
Medical Diagnosis: Saving Lives Through Early Detection
AI classification has made remarkable progress in medical imaging analysis. Deep learning models, particularly CNNs, now analyze radiological images to detect anomalies with accuracy comparable to – and sometimes exceeding – human specialists.
For example, Google Health developed a deep learning system that identifies diabetic retinopathy in eye scans with over 90% accuracy. The model analyzes subtle patterns in retinal images that might escape human detection, potentially preventing blindness through early intervention. Similarly, classification models now screen mammograms for signs of breast cancer, flagging suspicious areas for radiologist review and reducing both false negatives and physician workload.
These systems typically employ transfer learning, where networks pre-trained on general image datasets are fine-tuned on specialized medical images, allowing them to achieve high performance even with limited medical training data. Importantly, these tools augment rather than replace human expertise, acting as a “second opinion” that helps doctors make more informed decisions.
Fraud Detection: Protecting Financial Systems Through Pattern Recognition
Financial institutions deploy sophisticated classification models to identify fraudulent transactions among billions of legitimate ones. These systems must work in near real-time, balancing fraud prevention against customer convenience.
Modern fraud detection employs ensemble methods combining random forests, gradient boosting machines, and neural networks. These models analyze hundreds of features, including transaction amount, location, device information, and historical patterns. Feature engineering plays a crucial role, creating derived variables like “time since last transaction” or “deviation from typical spending pattern.”
FICO, a leading analytics company, estimates that AI classification models have helped prevent over $16 billion in credit card fraud annually in the United States alone. The most effective systems employ adaptive learning, continuously updating their understanding of fraud patterns as criminals develop new techniques.
Sentiment Analysis: Decoding Customer Emotions at Scale
Sentiment analysis applies classification to understand opinions expressed in text, categorizing customer reviews, social media mentions, and other communications as positive, negative, or neutral.
These systems typically employ natural language processing (NLP) techniques coupled with classification models. Modern approaches use Transformer-based models like BERT (Bidirectional Encoder Representations from Transformers) that capture contextual relationships between words, understanding nuances like sarcasm or implicit sentiment.
Major brands like Apple, Samsung, and Amazon analyze millions of customer comments daily to identify product issues, track brand perception, and prioritize improvements. Financial analysts also apply sentiment analysis to news articles and social media to gauge market sentiment about specific companies or economic trends, gaining insights that inform investment decisions.
Image Recognition: Enabling Visual Intelligence
Image recognition classification powers applications from mobile photo organization to autonomous vehicles. These systems primarily use CNNs to identify objects, people, scenes, and activities in visual data.
In retail, companies like Amazon employ image classification in their cashierless Amazon Go stores, automatically identifying products taken from shelves. Agricultural applications include crop disease detection, where farmers can photograph plants with their smartphones and receive immediate classification of potential diseases along with treatment recommendations.
Perhaps most significantly, autonomous vehicle systems rely on real-time image classification to identify pedestrians, vehicles, traffic signs, and road conditions. These systems must process visual data at high speeds with exceptional reliability, often combining multiple classification models with other sensing technologies.
Training and Evaluating AI Classification Models: A Practical Guide
Successful implementation requires a structured approach to model development and assessment. These practical steps help ensure robust, accurate classification systems.
Data Preprocessing and Feature Engineering: Building Strong Foundations
High-quality data preparation fundamentally determines classification success. This process includes several critical steps:
Data cleaning addresses missing values, outliers, and inconsistencies. Techniques range from simple imputation (replacing missing values with averages) to sophisticated approaches that maintain the statistical properties of the dataset.
Normalization and standardization ensure features exist on comparable scales, preventing models from overweighting variables simply because they have larger numerical values. Common techniques include min-max scaling and z-score normalization.
Feature selection and engineering identify the most informative variables and create new ones that help the model recognize important patterns. This might involve combining existing features, extracting temporal patterns, or creating categorical encodings.
For example, in a customer churn prediction system, raw data might include hundreds of customer attributes. Feature engineering might create new variables like “percentage decrease in usage over three months” or “days since last customer service contact” that more directly capture patterns relevant to churn risk.
Model Selection and Hyperparameter Tuning: Optimizing Performance
Choosing the right classification model involves balancing several considerations:
Problem complexity – Simpler problems with linear relationships might be effectively addressed with logistic regression, while complex image or text classification typically requires neural networks.
Available data – Deep learning models generally require substantial training data, while some traditional algorithms perform well with smaller datasets.
Interpretability requirements – Regulated industries often require explainable models, making decision trees or logistic regression preferable to “black box” approaches.
Computational constraints – Mobile applications or embedded systems may need lightweight models optimized for efficiency.
Once a model is selected, hyperparameter tuning optimizes its configuration. Techniques like grid search, random search, or Bayesian optimization systematically explore parameter combinations to identify optimal settings. This process typically employs cross-validation, where the model is trained and evaluated on different subsets of the data to ensure consistent performance.
Addressing Common Challenges in Classification Projects
Several challenges frequently arise in classification projects:
Imbalanced datasets, where some classes appear much more frequently than others, can lead models to ignore minority classes. Techniques like oversampling, undersampling, or synthetic minority oversampling (SMOTE) help address this issue by rebalancing class distributions.
Overfitting occurs when models learn training data too precisely, including its noise and peculiarities, leading to poor generalization. Regularization techniques, early stopping, and ensemble methods help mitigate this risk.
Data leakage happens when information from outside the training set influences model development, creating artificially high performance that doesn’t translate to real-world use. Careful cross-validation design and awareness of temporal data characteristics help prevent this problem.
The Future of AI Classification: Emerging Trends and Considerations
The field continues to evolve rapidly, with several important developments shaping its future direction.
Advancements in Deep Learning Architectures
Recent innovations in deep learning are expanding classification capabilities:
Few-shot learning techniques enable models to learn from very limited examples, potentially allowing classification in domains where labeled data is scarce or expensive to obtain.
Self-supervised learning approaches leverage unlabeled data to develop rich representations before fine-tuning on classification tasks, dramatically reducing the need for human-labeled examples.
Neural architecture search automates the design of neural network structures, potentially creating custom architectures optimized for specific classification problems without human intervention.
These technologies are reducing the barriers to implementing sophisticated classification systems across new domains and applications.
Explainable AI (XAI): Opening the Black Box
As classification models increasingly influence critical decisions, the demand for explainability has grown. Explainable AI techniques help humans understand how models reach their conclusions through:
Local interpretability methods like LIME (Local Interpretable Model-agnostic Explanations) and SHAP (SHapley Additive exPlanations) that explain individual predictions
Global interpretability approaches that reveal overall model behavior and feature importance
Inherently interpretable models designed for transparency from the ground up
Organizations like DARPA have launched major initiatives focused on XAI, recognizing its importance for adoption in sensitive domains like medicine, criminal justice, and financial services.
Ethical Considerations in Classification Systems
AI classification raises important ethical questions that responsible practitioners must address:
Bias and fairness concerns arise when models discriminate against certain groups, often reflecting biases in training data or problematic problem formulations. Techniques for fairness-aware machine learning seek to detect and mitigate these issues.
Privacy implications emerge when classification systems process sensitive personal data. Federated learning and differential privacy techniques allow models to learn from data without directly accessing it.
Accountability frameworks establish who bears responsibility for classification errors and how affected parties can seek recourse when automated systems make mistakes.
These considerations are increasingly incorporated into the entire model lifecycle, from problem definition through deployment and monitoring.
Conclusion: Selecting the Right AI Classification Approach
Throughout this comprehensive guide, we’ve explored the diverse landscape of AI classification training models and their real-world applications. Each model offers unique strengths suited to different challenges – from the interpretable simplicity of logistic regression to the powerful pattern recognition of deep neural networks.
The key to success lies not in choosing the most advanced or complex model, but in selecting the approach best aligned with your specific objectives, data characteristics, and operational constraints. Often, the most effective solutions combine multiple techniques in ensemble approaches that leverage the strengths of different models.
As AI classification continues to evolve, staying informed about emerging techniques while maintaining focus on fundamental principles will help you implement systems that deliver meaningful value. Whether you’re developing medical diagnostics, financial security systems, or customer experience enhancements, the thoughtful application of classification models can transform raw data into actionable insights.
We encourage you to experiment with these powerful tools, starting with simpler approaches before progressing to more complex models as your understanding and requirements grow. The rapidly expanding ecosystem of open-source libraries, pre-trained models, and educational resources makes AI classification more accessible than ever before.
External Resources:
About the author
Recent articles
Quad Core Dedicated Servers
Interested in Quad Core Dedicated Servers? View our inventory. What is a quad core dedicated...
Read More8 Core Dedicated Servers
For website owners looking to eliminate their hardware bottlenecks, the massive power capabilities of an...
Read MoreHow Unmetered Servers Can Help Businesses Grow
If you have a business website that is growing and expanding, it is essential that...
Read More